Implementation of artificial intelligence (AI) and machine learning in medical equipment
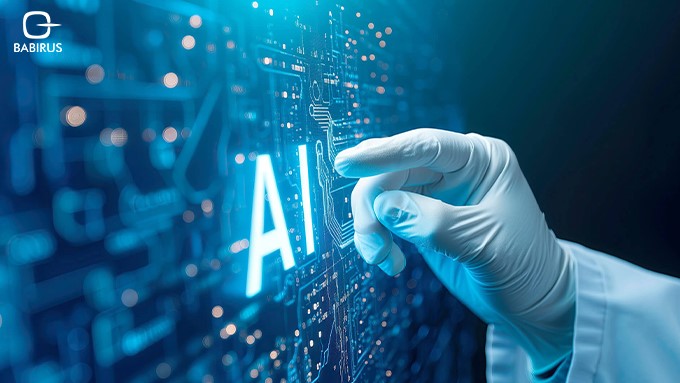
Imagine a world where medical devices not only assist but actively think, learn, and adapt to save lives. This isn’t a distant dream, it’s happening now. Artificial Intelligence (AI) and Machine Learning (ML) are transforming the healthcare industry, turning ordinary medical equipment into extraordinary tools for diagnosis, treatment, and patient monitoring.
Continue reading as we dive into the exciting world of medical AI technology, exploring its applications, benefits, and challenges of AI in medical equipment, exploring how machine learning in healthcare is shaping the future of the medical AI technology industry.
What is AI and Machine Learning in Healthcare?
Artificial Intelligence (AI) and Machine Learning (ML) are transforming healthcare by allowing machines to simulate human decision-making and adapt through data analysis. While AI encompasses the broader capability of machines to process information, ML is a specialized branch where algorithms learn and improve autonomously by analyzing patterns and making data-driven decisions.
Artificial Intelligence and Machine Learning encompass various approaches, each contributing uniquely to healthcare innovation. Here’s an exploration of the key types and their applications:
Supervised Learning: Key Insights for Healthcare AI
Supervised learning involves training algorithms on labeled datasets, where input-output pairs are predefined. The system learns from this data to make accurate predictions or classifications. For instance, supervised learning is widely used in AI diagnostic tools to detect abnormalities in medical imaging. Algorithms trained on labeled datasets can identify tumors in CT scans or recognize irregularities in heart rhythm through ECG analysis, ensuring precise and reliable results.
Exploring Unsupervised Learning in Medical AI
Unlike supervised learning, unsupervised learning works with unlabeled datasets. The algorithm identifies hidden patterns or clusters within the data without pre-defined categories. In healthcare, unsupervised learning is instrumental in machine learning in healthcare for tasks like grouping patients with similar symptoms or discovering unknown correlations in genetic data. These insights can lead to personalized treatment plans and a deeper understanding of complex diseases.
How Reinforcement Learning Enhances Medical Robotics
Reinforcement learning is a dynamic learning process where algorithms improve through trial and feedback, optimizing performance over time. This type of learning is particularly valuable in surgical robotics. For example, robotic systems can analyze data from previous surgeries to enhance precision and adapt to varying surgical scenarios. Reinforcement learning’s ability to evolve makes it critical for advancing autonomous decision-making in medical technologies.
NLP in Healthcare: Transforming Medical Data Analysis
Natural Language Processing enables AI to interpret and process human language, bridging the gap between unstructured text and actionable insights. In healthcare, NLP powers medical AI technology by extracting meaningful data from electronic health records (EHRs), patient feedback, or research papers. Applications include summarizing medical history, automating administrative tasks, and analyzing sentiment in patient reviews to improve care strategies. By processing vast amounts of unstructured data, NLP enhances decision-making and streamlines healthcare workflows.
Deep Learning in Healthcare: A Comprehensive Guide
Deep learning utilizes advanced neural networks modeled after the human brain to solve intricate problems. Its layered architecture processes data hierarchically, making it particularly effective for complex tasks. In medical imaging, deep learning is used to analyze high-resolution scans, identifying subtle indicators of diseases like diabetic retinopathy or early-stage cancer. It is also employed in predictive modeling, such as estimating disease progression based on patient history. Deep learning’s ability to process complex datasets drives innovation across the AI in healthcare industry, from diagnostics to therapeutic planning.
Real-World Applications of AI in Medical Equipment
AI in medical equipment and machine learning in healthcare are enhancing diagnostic accuracy, streamlining operations, and enabling more personalized care for patients. With these advancements, the integration of AI in medical devices has expanded into various applications like:
Revolutionizing Medical Imaging with AI Technology
AI diagnostic tools have significantly improved the accuracy and speed of interpreting medical images. Dubai as a hub for medical equipment innovation supports this progress, helping radiologists detect conditions with greater accuracy. Advanced algorithms can identify anomalies in X-rays, MRIs, and CT scans, assisting radiologists in detecting conditions like fractures, tumors, and vascular diseases at an early stage.
AI in Wearable Health Devices and Remote Monitoring
Machine learning in healthcare has made wearable devices more efficient in monitoring patient vitals such as heart rate, oxygen levels, and glucose levels. AI in medical equipment like smartwatches and remote monitoring systems empowers patients to manage their health proactively while providing real-time data to healthcare providers.
The Role of AI in Next-Gen Surgical Robotics
AI-powered surgical robots are changing operating rooms. With precision and data-driven decision-making, these systems assist surgeons in performing minimally invasive procedures, reducing recovery times and improving patient outcomes.
AI-Driven Drug Discovery and Development Processes
Medical AI technology is accelerating the process of drug discovery by analyzing vast datasets to identify potential compounds and predict their efficacy. Machine learning models are also helping optimize clinical trials, making the process faster and more cost-effective.
Advantages of AI and Machine Learning in Healthcare
- Enhanced Diagnostic Accuracy: Algorithms trained on vast datasets can identify patterns that may not be apparent to the human eye, improving the precision of diagnoses.
- Personalized Treatment Plans: AI-powered systems analyze individual patient data to recommend tailored therapies, enhancing treatment outcomes.
- Operational Efficiency: Automation of administrative tasks, from scheduling to billing, reduces the workload on healthcare staff and minimizes errors.
- Proactive Health Management: AI and machine learning tools enable early detection of diseases, allowing for timely interventions and reducing healthcare costs.
Challenges and Ethics in AI-Driven Medical Solutions
While the integration of AI in medical equipment offers numerous benefits, it also comes with significant challenges and ethical concerns that need to be addressed for its safe and effective application in healthcare.
Ensuring Data Privacy and Security in AI Healthcare
AI in healthcare industry relies on vast datasets, often containing sensitive patient information. This dependence raises critical concerns about data breaches and unauthorized access, which could erode trust in AI-driven systems. Ensuring robust encryption, regular system audits, and adherence to stringent data protection regulations can mitigate these risks. Moreover, implementing frameworks like multi-factor authentication and real-time intrusion detection systems further fortifies security in medical AI technology.
Addressing Bias and Fairness in AI Medical Tools
Bias in AI systems arises when training datasets fail to represent the full spectrum of patient demographics, leading to skewed outcomes that can exacerbate health disparities. For example, diagnostic tools trained on data from specific populations may yield inaccurate results for underrepresented groups. Developing diverse datasets and conducting regular bias audits are essential steps to address these disparities. AI developers must also employ fairness metrics and engage with healthcare professionals to ensure equitable outcomes for all patients.
Overcoming Regulatory Barriers in AI Technologies
AI diagnostic tools and machine learning in healthcare must undergo regulatory hurdles to ensure safety and reliability. However, the rapid evolution of AI technologies often outpaces existing regulations, causing delays in approvals. Governments and regulatory bodies can address this by establishing adaptive policies that evolve alongside technological advancements, such as creating regulatory sandboxes for testing AI solutions under controlled conditions.
Demystifying AI: The Explainability Challenge
Some AI systems, especially those based on deep learning, operate as “black boxes,” meaning their decision-making processes are difficult to understand. This lack of transparency can lead to hesitation among clinicians and patients alike. To build trust, developers are exploring ways to make AI decisions more interpretable. For example, tools that highlight the areas of a medical image influencing a diagnosis can provide clinicians with clarity and confidence in AI-supported recommendations.
The Importance of Human-AI Collaboration in Medicine
AI systems are powerful, but they are not a replacement for human expertise. Over-reliance on AI can lead to important details being missed, especially in complex medical cases. To prevent this, AI should be seen as a tool to support, not replace, clinical judgment. Comprehensive training for healthcare professionals can ensure AI is used effectively as part of a broader diagnostic and treatment strategy.
Key Ethical Concerns for AI in Healthcare
AI in medical equipment raises questions about accountability and patient autonomy. For example, who is responsible if an AI system provides a flawed diagnosis or recommendation? To address these concerns, healthcare organizations should develop clear guidelines that define the role of AI in patient care. Involving ethicists and clinicians in these discussions ensures AI use aligns with broader medical and societal values.
Future Trends in AI and Machine Learning in Medicine
The incorporation of AI in medical equipment continues to reshape the healthcare industry, opening doors to innovative solutions and enhanced patient outcomes. As technology advances, these developments are expected to address challenges and create new opportunities for improved healthcare delivery. Here are the emerging trends shaping the future of AI in medical equipment:
Personalized and Precision Medicine
AI-powered devices are driving a shift toward precision medicine, where treatments are tailored to each patient’s unique needs. Machine learning algorithms analyze diverse data sources, such as genetics, lifestyle, and medical history, to recommend therapies that are both targeted and effective. For example, wearable devices integrated with AI can monitor health metrics in real time and make automatic adjustments to treatments, such as optimizing insulin delivery for diabetics.
IoT Integration for Enhanced Monitoring
The Internet of Things (IoT) is transforming healthcare by connecting medical devices into a cohesive system that allows for real-time monitoring and data sharing. Devices with AI and IoT functionality; such as wearable health trackers and implantable sensors, enable continuous health surveillance. These systems notify patients and providers instantly in case of anomalies, helping prevent emergencies and promoting proactive care for chronic conditions.
AI in Advanced Surgical Tools
Surgical robotics powered by AI are advancing to handle increasingly complex procedures with greater precision and reliability. Future systems will not only assist surgeons in performing minimally invasive procedures but also offer real-time analytics, suggest optimized techniques, and reduce the likelihood of errors. These advancements promise better outcomes and quicker recovery times for patients.
Predictive Analytics for Early Detection
AI diagnostic tools are being developed to predict diseases before symptoms appear. Using data from patient histories and other risk factors, these systems analyze patterns to flag potential health issues early. Early intervention allows healthcare providers to mitigate risks and enhance outcomes, particularly for conditions such as cardiovascular diseases and cancers.
Innovations in Medical Imaging
AI systems are advancing medical imaging by improving clarity and reducing radiation exposure. AI-based imaging tools help radiologists detect subtle signs of disease that might otherwise go unnoticed. This innovation is particularly beneficial for conditions requiring regular imaging, such as cancer monitoring and chronic lung diseases.
Collaborative AI for Clinician Support
Future systems will emphasize collaboration between AI and healthcare professionals. Rather than replacing human expertise, these systems will offer data-driven insights and recommendations, allowing clinicians to make informed decisions. Collaborative AI aims to enhance care delivery while maintaining the critical human connection in healthcare.
Lastly,
Artificial intelligence and machine learning are changing how we think about medical equipment in healthcare. They’re making diagnostics more accurate, treatments more personalized, and processes easier for everyone involved. Of course, challenges like keeping data safe and addressing ethical concerns still need attention, but the possibilities these technologies bring are exciting. As AI in medical equipment continues to grow, it’s paving the way for a healthcare system that truly prioritizes the needs of both patients and providers.